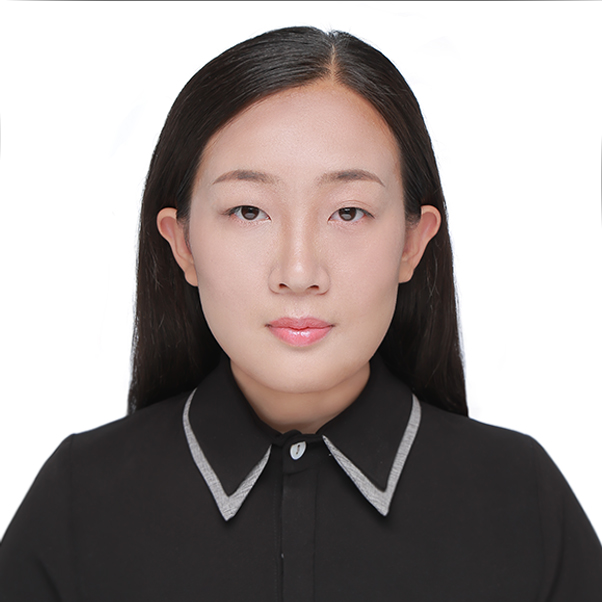
Shuting Shen
Postdoctoral fellow at Fuqua School of Business and Department of Biostatistics & Bioinformatics, Duke University
Research Interest
I am a postdoctoral fellow at the Fuqua School of Business and the Department of Biostatistics & Bioinformatics at Duke University, jointly supervised by Dr. Alexandre Belloni and Dr. Ethan X. Fang. Prior to my postdoctoral position, I obtained my PhD in Biostatistics from Harvard University, where I was fortunate to be jointly supervised by Dr. Xihong Lin and Dr. Junwei Lu. My research interests primarily include large-scale inference, combinatorial inference, choice model asymptotics, operations research theories, applied probability, and distributed computing.
Email
ss1446@duke.edu
Selected Papers
Alexandre Belloni, Ethan X. Fang, Shuting Shen (alphabetical order)
We derive novel anti-concentration bounds for the difference between the maximal values of two Gaussian random vectors across various settings. Our bounds are dimension-free, scaling with the dimension of the Gaussian vectors only through the smaller expected maximum of the Gaussian subvectors. In addition, our bounds hold under the degenerate covariance structures, which previous results do not cover. In addition, we show that our conditions are sharp under the homogeneous component-wise variance setting, while we only impose some mild assumptions on the covariance structures under the heterogeneous variance setting. We apply the new anti-concentration bounds to derive the central limit theorem for the maximizers of discrete empirical processes. Finally, we back up our theoretical findings with comprehensive numerical studies.
Dimension Reduction for Large-Scale Federated Data: Statistical Rate and Asymptotic Inference Under revision at Journal of the American Statistical Association, Theory and Methods
Shuting Shen, Junwei Lu, and Xihong Lin
Principal component analysis (PCA) is one of the most popular methods for dimension reduction. In light of rapidly increasing large-scale data in federated ecosystems, where data cannot leave individual warehouses, such as banks and healthcare systems, the traditional PCA method is often not applicable due to privacy protection considerations and large computational burden. Fast PCA algorithms have been proposed to lower the computational cost for large-scale data, but they cannot handle federated data. Distributed PCA algorithms have been developed to handle federated data by applying traditional PCA to data at each site and aggregating site-specific PCA results. However, they are not computationally efficient and not scalable when data at each site are large with many samples and variables, such as biobanks. In this paper, we propose the FAst DIstributed (FADI) PCA method that performs PCA analysis of large federated data with high computational efficiency and low statistical error without the need of sharing the data across sites. Specifically, FADI applies fast PCA to site-specific data using multiple random sketches and aggregates the fast PCA results across sites. We perform a non-asymptotic theoretical study to show that under some regularity conditions, FADI enjoys the same error rate as the traditional full sample PCA and a significantly smaller order of computational burden compared to the existing methods. We perform extensive simulation studies to compare the finite sample performance of FADI with the existing algorithms, and show that FADI substantially outperforms the other methods in computational efficiency without sacrificing statistical accuracy. We apply FADI to the analysis of the 1000 Genomes data to study the population structure.
Combinatorial Inference on the Optimal Assortment in the Multinomial Logit Model Under revision at Operations Research (abstract at EC'23 )
Shuting Shen, Xi Chen, Ethan X. Fang, Junwei Lu
Assortment optimization has received active explorations in the past few decades due to its practical importance. Despite the extensive literature dealing with optimization algorithms and latent score estimation, uncertainty quantification for the optimal assortment still needs to be explored and is of great practical significance. Instead of estimating and recovering the complete optimal offer set, decision-makers may only be interested in testing whether a given property holds true for the optimal assortment, such as whether they should include several products of interest in the optimal set, or how many categories of products the optimal set should include. This paper proposes a novel inferential framework for testing such properties. We consider the widely adopted multinomial logit (MNL) model, where we assume that each customer will purchase an item within the offered products with a probability proportional to the underlying preference score associated with the product. We reduce inferring a general optimal assortment property to quantifying the uncertainty associated with the sign change point detection of the marginal revenue gaps. We show the asymptotic normality of the marginal revenue gap estimator, and construct a maximum statistic via the gap estimators to detect the sign change point. By approximating the distribution of the maximum statistic with multiplier bootstrap techniques, we propose a valid testing procedure. We also conduct numerical experiments to assess the performance of our method.
Combinatorial-Probabilistic Trade-Off: P-Values of Community Properties Test in the Stochastic Block Models IEEE Transactions on Information Theory, Vol. 69, NO. 10, October 2023 (short version is ICLR 2023 spotlight)
Shuting Shen, Junwei Lu
In this paper, we propose an inferential framework testing the general community combinatorial properties of the stochastic block model. We aim to test the hypothesis on whether a certain community property is satisfied and provide p-values to assess the statistical uncertainty. For instance, we test whether a given set of nodes belong to the same community. We present a general framework applicable to all symmetric community properties. To ease the challenges caused by the combinatorial nature of communities properties, we develop a novel shadowing bootstrap testing method. Utilizing the symmetry, our method can find a shadowing representative of the true assignment and the number of assignments to be tested in the alternative can be largely reduced. In theory, we introduce a combi- natorial distance between two community classes and show a combinatorial-probabilistic trade-off phenomenon. Our test is honest as long as the product of the combinatorial distance between two communities and the probabilistic distance between two assignment probabilities is sufficiently large. Besides, we show that such trade-off also exists in the information-theoretic lower bound of the community property test. We also implement numerical experiments on both the synthetic data and the protein interaction application to show the validity of our method.
Honors & Awards
ASA SLDS Student Paper Award
2023
WNAR Best Student Paper Award
2022
ICSA Junior Research Award
2022
ICSA Student Paper Award
2022
NESS Student Research Award
2022
Robert B. Reed Prize for Excellence in Biostatistical Science
Harvard University | 2020
The Robert Balentine Reed Prize for Excellence in Biostatistical Science is awarded each year to the student(s) receiving the highest grade on the Department’s written qualifying exam.
1st Prize (Provincial), National High School Math League
2012